Electron microscopy is one of the most important methods for studying the micro- and nano-scale morphology of catalysts, materials, and biological objects. However, any applications of the method require collecting large amounts of statistically-significant data, significantly complicating its acquisition and analysis. In the talk, solutions to these problems will be described. It will be shown high automated data acquisition can be performed hierarchically, enabled by unsupervised machine learning algorithms. Multiple examples for the use of deep learning algorithms in electron microscopy data analysis will be presented.
Watch a recording of the presentation below:
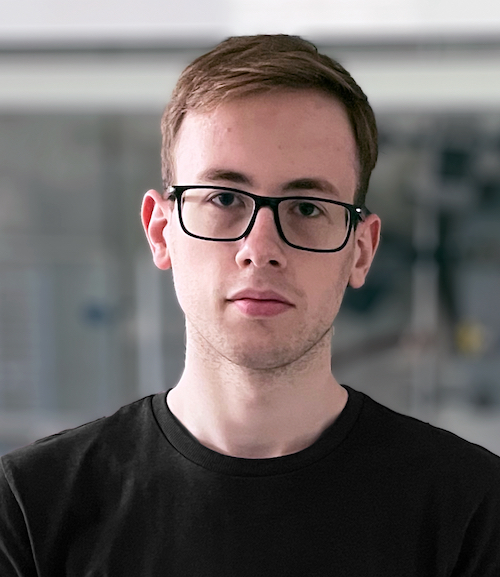
Biography
Daniil Boiko received his MSc degree in organic chemistry at Lomonosov Moscow State University. He worked in prof. Valentine Ananikov’s group, leading efforts in discovering novel applications of machine learning methods in chemistry. In particular, he is interested in combining machine learning with electron microscopy and mass-spectrometry, as well as in reaction discovery. He is currently doing a PhD in Chemical Engineering at Carnegie Mellon University.