Numerous disciplines, such as image recognition and machine translation, have been revolutionized by using machine learning (ML) to leverage big data. In organic synthesis, providing accurate chemical reactivity prediction with ML models could assist chemists with reaction prediction, optimization, and mechanistic interrogation. This talk will cover the Doyle groupās efforts on experimental data collection and the quest to expand its availability and limit its bias for data science applications; feature engineering that may extend common intuition about the underlying chemistry; model assessments in the regime of small to medium size reaction datasets; and opportunities arising from accurate model predictions and their mechanistic interpretation.
Biography
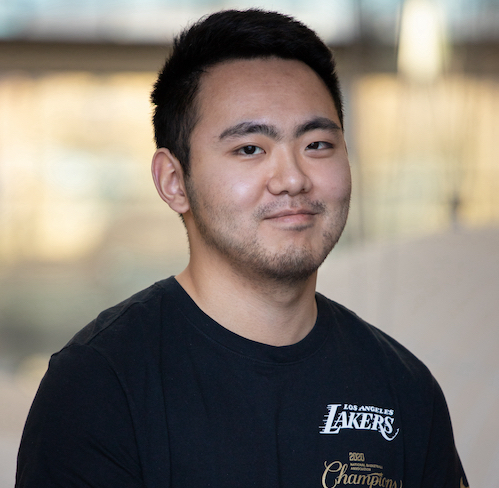
Jason is a third year PhD student in the Doyle group. He did undergraduate research in the Rovis group at Columbia University and graduated with a B.A. in biochemistry and B.A. in computer science. After that, he moved to Princeton and subsequently UCLA to pursue a PhD in chemistry. His research focuses on the application of machine learning models in synthetic chemistry.Ā
Watch a recording of the presentation below: